The human sense of hearing is a highly-developed system: when we want to follow a conversation, we can block out disturbing background noise. At the same time, we perceive alarming noises immediately even under noisy conditions. We can predict with a good degree of accuracy the direction from which a noise is coming and how far away the source of the sound is. And all this with only two ears as sound receivers. In fact, most of the work actually takes place between the ears. In order to teach computers to hear, scientists from the Division for Hearing, Speech and Audio Technology at the Fraunhofer IDMT work together with scientists conducting fundamental research at the University of Oldenburg to develop computer models that simulate the human sense of hearing. The application fields for the technology extend from Smart Home and Smart City through to Industry 4.0. Interview with Dr.-Ing. Stefan Goetze
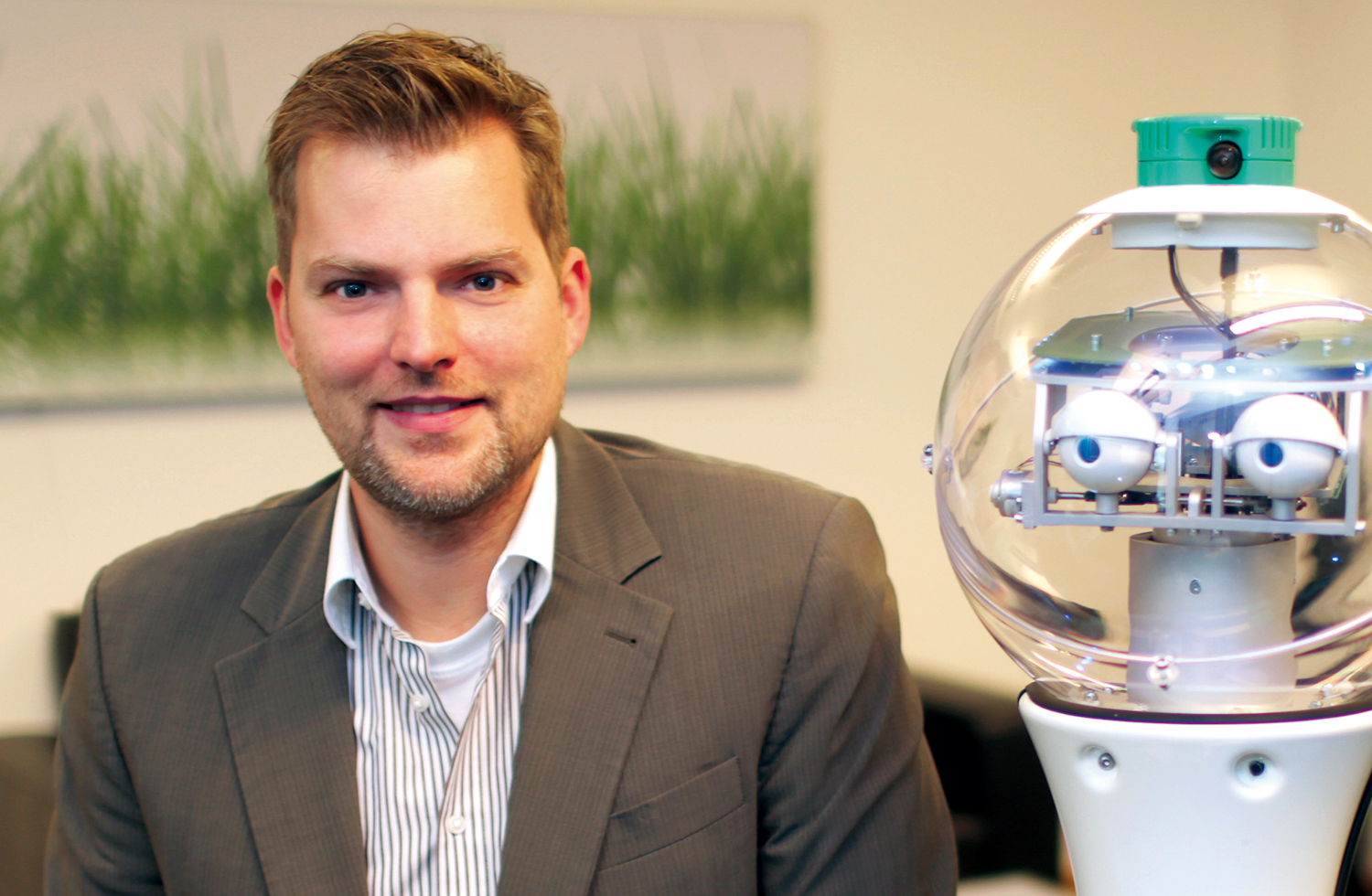
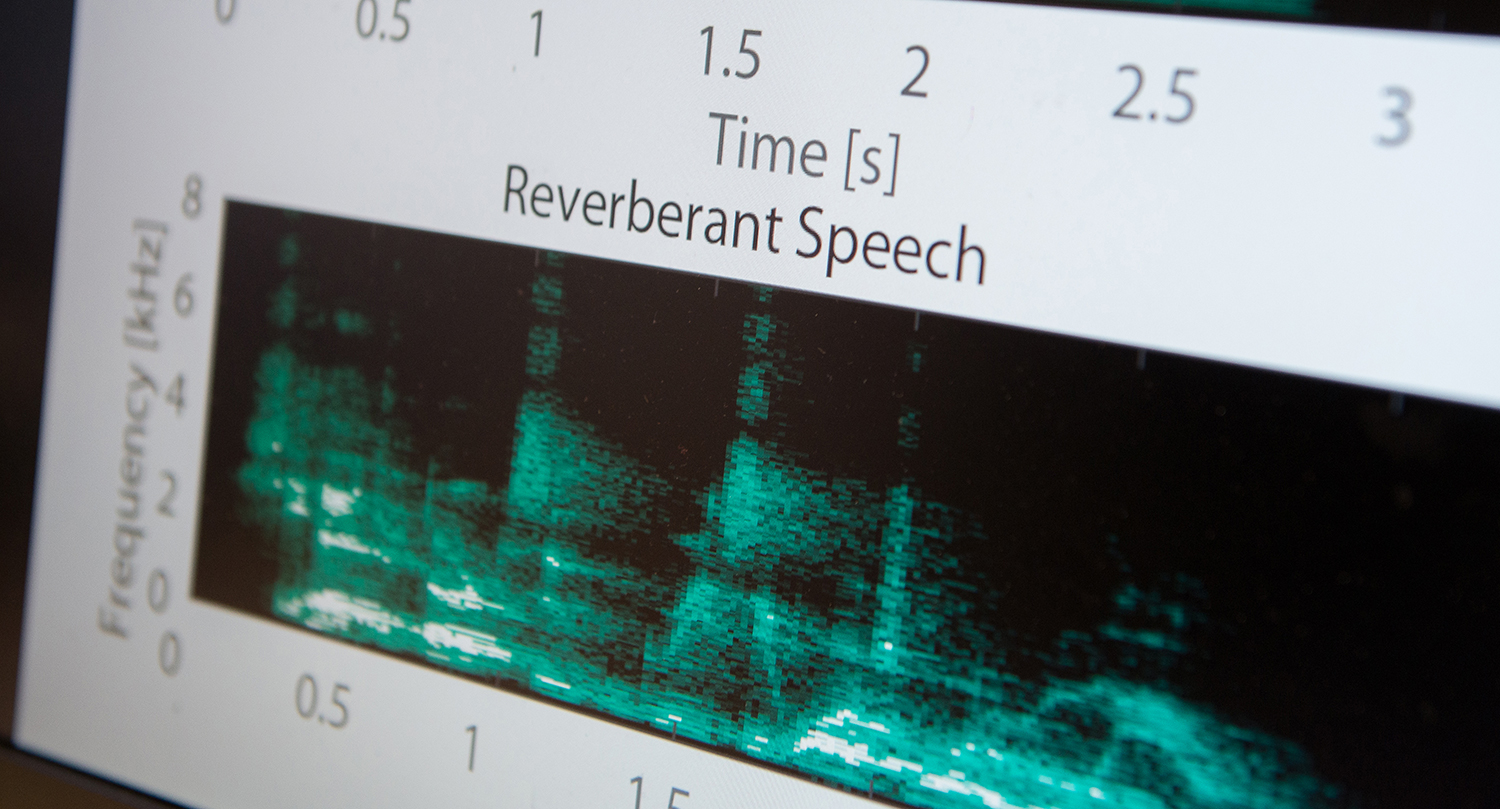
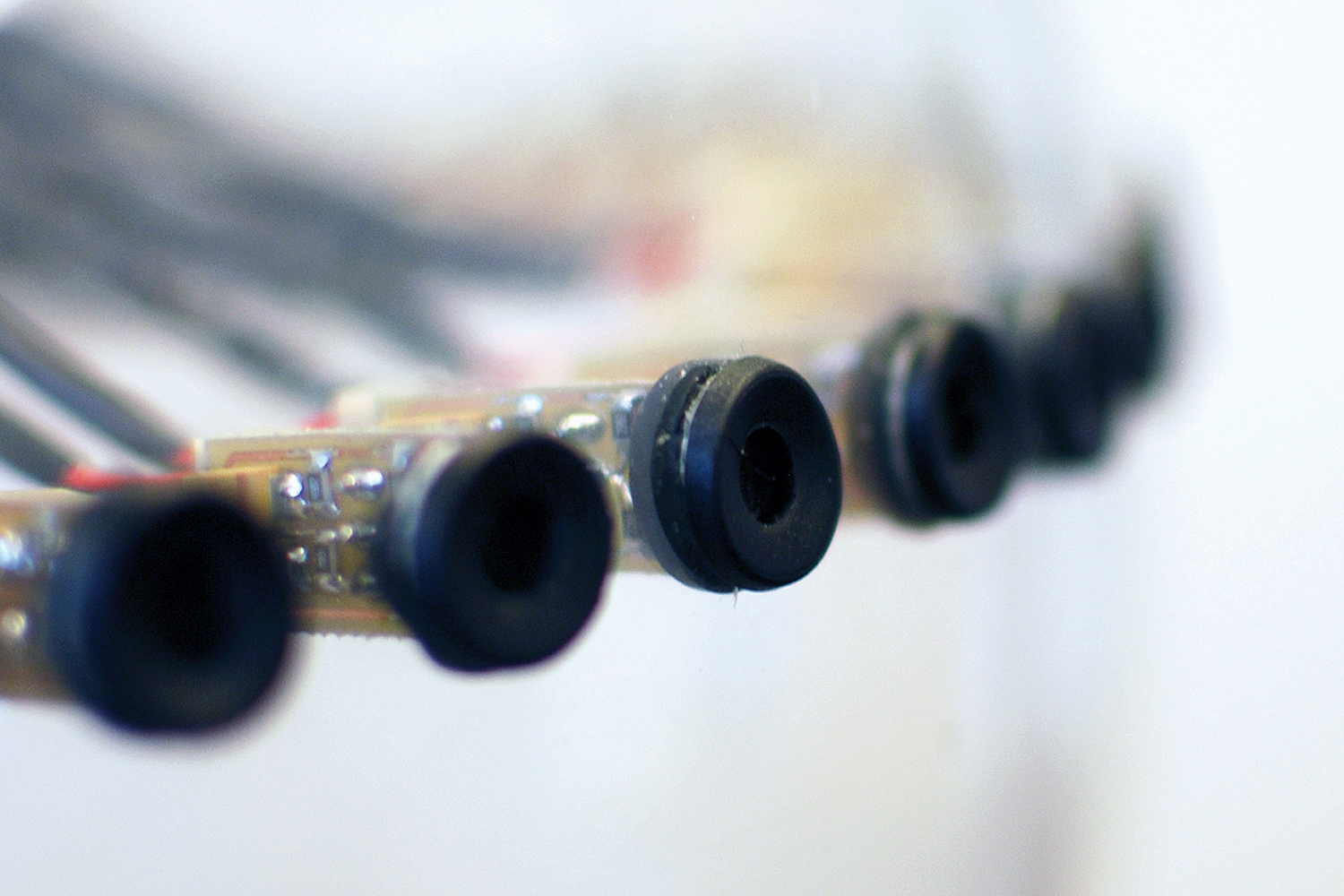
Stefan, in your research group you teach computers to hear – how do you do that?
The basis for our work is provided by computer models that simulate human hearing perception. Using algorithms that describe speech and noises, we develop machine learning methods in order to teach computers to recognise acoustic events. Our recogniser systems are trained with many hours of audio material. The audio signal is converted for this into so-called acoustic characteristics, which the computer then recognises as patterns. In our project group we have created a database with hundreds of different characteristics which are based on the results of fundamental research on hearing. We apply both statistical techniques and methods from the field of artificial intelligence for computer-based pattern recognition.
How well do these methods work?
At the moment, the best recognition results are obtained with so-called artificial neural networks – that is to say algorithms which emulate the processing of signals in the human brain. We get close here to human recognition performance for recognition of non-speech acoustic events. However, this is also only around 80 percent in human beings if the visual sense is not used at the same time. For speech recognition, the recogniser performance must be around 95 percent to be acceptable. However, avoidance of incorrect recognition is even more important: for example, if the speech recognition system in a Smart Home interprets a snoring noise incorrectly and switches on the light, the acceptance level for such a technical system will be practically zero.
Speech recognition systems are often unreliable particularly in conditions with background noise or when the microphone is too far away from the speaker – anyone who has used the speech recognition function in their smartphone or with a car navigation system is familiar with this problem. How can that be improved?
Room reverberation and interference noise can considerably influence the perception of speech and music. This problem also exists for computer-based hearing. Every room has different acoustic characteristics which can change the sound in an unpredictable way. One of the main research areas of our group is development of algorithms that reduce reverberation and interference noise in order to improve the intelligibility of speech. Systems for automatic speech and event recognition function much more robustly with intelligent signal preprocessing. Our algorithms are able to distinguish and filter out speech and defined acoustic events from reverberation and interference noise.
Humans have two ears – how many microphones does a computer need to “hear”?
At least two sound receivers are needed for spatial hearing. The human brain calculates the time offset and the difference in intensity between the sound waves arriving at the left and right ears in order to locate the source of a noise. We use several microphones, so-called microphone arrays, if spatial information is important for the recognition process. Depending on the application scenario, we use very tiny and also up to a hundred microphones. On the basis of these sound sources, a computer program, for example, can calculate the direction from which speaking is taking place and suppress noises from other directions. This method is called “beamforming”. Beamforming makes it possible to focus on a noise source and to zoom it up close.
Stefan, why are you teaching computers to hear?
Acoustic speech and event recognition can be used in an extremely wide range of everyday technologies – from speech recognition in a smartphone or Smart Home or security applications in Smart Cities through to acoustic monitoring of production machines. One of our first projects was acoustic differentiation between dry and productive coughing. Together with our industry partners, we then developed intelligent emergency call systems for inpatient and home care. These systems are able to acoustically detect not just cries for help, but also critical events such as whimpering or breaking glass. As part of international research projects, we have since further investigated the potential of this technology in different scenarios. Such scenarios include traffic monitoring or detection of security-critical events in Smart Cities, for example. We are currently studying the potential of recogniser systems in Industry 4.0 scenarios.